As the affiliate marketing industry continues to grow, AI-driven fraud Detection Tools For Affiliate Marketing are becoming essential in the fight against fraud. Marketers must adopt proactive measures to protect their investments with billions of dollars at stake.
In this in-depth article, we’ll explore into the world of AI-driven fraud detection tools, uncovering their advantages and disadvantages, exploring compelling case studies, and revealing much more to empower your understanding and decision-making.
Introduction To AI-Driven Fraud Detection Tools
AI-driven fraud detection tools leverage advanced algorithms and machine learning techniques to analyze vast amounts of data in real-time, identifying suspicious activities that traditional methods might overlook.
These tools can detect fraud patterns, such as click fraud, affiliate impersonation, and transaction anomalies, providing marketers with actionable insights to combat these threats effectively.
Integrating these AI solutions into their marketing strategies, affiliates can safeguard their revenue and foster trust and transparency within their partnerships, ultimately leading to sustainable growth in an increasingly competitive marketplace.
What Are AI-Driven Fraud Detection Tools?
AI-driven fraud detection tools are software solutions that utilize artificial intelligence and machine learning algorithms to identify and prevent fraudulent activities in various industries, including affiliate marketing.
These tools analyze vast amounts of data from transactions, user behavior, and other relevant sources in real-time to detect anomalies and patterns indicative of fraud.
Key features of AI-driven fraud detection tools include:
- Real-Time Monitoring: Continuous analysis of transactions and activities to detect suspicious behavior as it occurs.
- Pattern Recognition: Machine learning algorithms learn from historical data to identify common fraud patterns and adapt to new tactics used by fraudsters.
- Anomaly Detection: The ability to flag unusual activities that deviate from established user behavior or transaction norms.
- Automated Alerts: Immediate notifications for marketers when potential fraud is detected, allowing for prompt action.
- Data Integration: The capability to aggregate data from multiple sources, providing a comprehensive view of user behavior and transaction history.
- Predictive Analytics: Using historical data to predict future fraud risks and trends, enabling proactive measures.
Here’s A List Of AI-Driven Fraud Detection Tools That Are Widely Recognized
1. SAS Fraud Management
- Operation: SAS Fraud Management integrates with existing systems to monitor transactions in real-time. It uses advanced analytics, including machine learning and artificial intelligence, to detect unusual behavior and patterns that could indicate fraudulent activity. The system is designed to analyze large volumes of data quickly and accurately, allowing businesses to respond to potential threats almost instantaneously.
- Industries: SAS Fraud Management benefits the most from financial services, insurance, telecommunications, and retail sectors, given its ability to process vast amounts of transaction data.
- Unique Technologies: The tool employs predictive modeling, which anticipates future fraud based on historical data, and behavioral analytics, which understands normal versus suspicious behavior within transactional data.
2. FICO Falcon
- Operation: FICO Falcon combines transaction pattern analysis and customer profiling. It creates a unique profile for each customer and monitors their behavior over time. Any deviation from established patterns triggers a fraud alert. FICO Falcon’s AI engine continuously learns and adapts to new fraud tactics, enhancing its detection capabilities over time.
- Industries: Primarily used in banking, credit card companies, and financial institutions. It’s particularly effective in identifying credit card fraud, unauthorized transactions, and account takeovers.
- Unique Technologies: FICO Falcon’s machine-learning algorithms can process billions of transactions in real time, enabling financial institutions to prevent fraud before it affects their customers. Its predictive analytics also reduce the occurrence of false positives, making it a reliable tool for large-scale operations.
3. Fraud.net
- Operation: Fraud.net is a cloud-based platform that uses AI to aggregate data from various sources, including transactions, customer behaviors, and historical fraud cases. It then applies machine learning algorithms to detect anomalies and flag potentially fraudulent activities.
- Industries: E-commerce, travel, and hospitality industries frequently use Fraud.net, as these sectors often deal with large volumes of online transactions where fraud risks are high.
- Unique Technologies: Fraud.net’s AI-powered risk scoring assesses each transaction’s likelihood of being fraudulent. Additionally, its cross-channel analysis ensures consistent fraud prevention across multiple customer interaction points, such as online, mobile, and in-store channels.
4. Kount
- Operation: Kount’s AI-driven platform analyzes digital signals, device fingerprinting, and user behaviors to detect fraud. It leverages a vast global network of fraud data to identify and mitigate threats in real time. The system continuously updates its algorithms to recognize new fraud patterns and tactics.
- Industries: Kount’s capabilities benefit e-commerce, retail, and payment service providers greatly, particularly in reducing chargebacks and preventing payment fraud.
- Unique Technologies: Kount’s Identity Trust Global Network uses machine learning to create a digital identity for each user, which is then tracked across devices and transactions. This comprehensive approach helps businesses detect fraud even if the same fraudster uses a new device or IP address.
5. Darktrace
- Operation: Darktrace’s AI, known as the Enterprise Immune System, mirrors the human immune system by learning what is normal for each network it monitors. It then identifies deviations from this norm, flagging them as potential threats. The system can autonomously respond, automatically neutralizing threats before they can cause harm.
- Industries: Healthcare, finance, and critical infrastructure sectors often deploy Darktrace to protect sensitive data and ensure compliance with regulations.
- Unique Technologies: Darktrace uses unsupervised machine learning, meaning it doesn’t rely on historical data to identify threats. Instead, it learns in real time, adapting to the unique characteristics of the network it’s protecting. This makes it particularly effective against novel threats and zero-day exploits.
6. Acuant AssureID
- Operation: Acuant AssureID specializes in identity verification by scanning and analyzing government-issued IDs, passports, and other documents. It uses AI to detect forgeries and inconsistencies, ensuring the person presenting the ID is who they claim to be.
- Industries: It is widely used in the financial services, healthcare, and travel industries, where verifying a person’s identity is crucial for both security and compliance purposes.
- Unique Technologies: Acuant’s AI-powered document verification process is enhanced by biometric analysis, which compares the person’s face to the photo on their ID. The tool can also detect microprints, holograms, and other security features embedded in documents to prevent forgery.
7. Emailage
- Operation: Emailage assesses the risk associated with email addresses used in transactions. By analyzing the domain, the age of the email address, and its history in prior transactions, Emailage can predict the likelihood of fraud.
- Industries: E-commerce, banking, and online marketplaces often use Emailage to prevent fraud during account creation and transaction processes.
- Unique Technologies: The tool’s global intelligence network aggregates data from billions of email addresses, allowing it to provide a comprehensive risk score for each transaction. It’s particularly effective against phishing attacks and fraud attempts from newly created or compromised email addresses.
8. Zensed
- Operation: Zensed uses AI to analyze transactions in patterns and behaviors indicative of fraud. It allows businesses to create custom rules and filters, tailoring the fraud detection process to their specific needs.
- Industries: Small to medium-sized e-commerce businesses and startups often use Zensed for its flexibility and scalability.
- Unique Technologies: Zensed’s machine learning algorithms are designed to minimize false positives while accurately detecting fraud. The platform’s real-time processing ensures businesses can react quickly to suspicious activity, reducing the risk of revenue loss.
Enter the hero of our story: AI-driven fraud detection tools are nifty tech that are reshaping the battle against fraud, making it easier for marketers to spot and stop shady activities before they wreak havoc on their campaigns.
Here’s a Representation Of A Table Graph Ranking AI-driven Fraud Detection Tools By Popularity And Price:
Tool Name | Popularity Score | Price (USD per year) |
---|---|---|
SAS Fraud Management | 85 | 15,000 |
Fraud.net | 78 | 10,000 |
Kount | 88 | 12,000 |
Darktrace | 92 | 18,000 |
Acuant AssureID | 76 | 8,000 |
Emailage | 80 | 9,000 |
Zensed | 70 | 7,000 |
FICO Falcon | 90 | 20,000 |
Why AI-Driven Fraud Detection Tools For Affiliate Marketing:
Now, you might wonder why AI? Traditional fraud detection methods often rely on manual processes, which are time-consuming and prone to errors.
Conversely, AI leverages machine learning and data analytics to identify unusual patterns and behaviors with pinpoint accuracy. It’s like having a super-smart detective on your team who never sleeps.
In the age of digital transformation, AI-driven tools are becoming indispensable for affiliate marketers.
They simplify processes, enhance accuracy, and, most importantly, protect both advertisers and affiliates from fraud.
Plus, they relieve human analysts from the mundane task of sifting through mountains of data, allowing them to focus on strategic planning and decision-making.
AI is giving affiliate marketing a new lease on life. By embracing these tools, businesses can safeguard their campaigns, improve ROI, and build stronger, more trustworthy relationships with their affiliates.
In-depth Key Features of AI-Driven Fraud Detection Tools
AI-driven fraud detection tools come packed with features that make them indispensable for modern affiliate marketers.
Each feature enhances security, accuracy, and ease of use, ensuring your campaigns are practical and fraud-free.
Machine learning algorithms are the backbone of these tools. Machine learning can identify anomalies that indicate fraudulent activity by analyzing vast amounts of data.
AI learning continuously learns and adapts unlike manual methods, making it more accurate. Think of it as a tireless watchdog that’s always on alert.
Real-time monitoring and alerts are another game-changer. The system notifies you instantly the moment suspicious activity is detected.
This allows for rapid response, minimizing damage and protecting your revenue. Alerts can be customized based on specific criteria, ensuring you get relevant and actionable information.
Behavioral analysis and pattern recognition take things a step further. These features analyze user behavior over time, identifying patterns that deviate from the norm.
It’s like having a magnifying glass on each click, conversion, and interaction, helping you detect even the most subtle forms of fraud.
Integration with existing marketing platforms is also crucial. These AI tools can easily be integrated into your current setup and work seamlessly with the software you’re already using.
This means no complicated overhauls or steep learning curves—just a smooth transition into enhanced fraud protection.
So, machine learning algorithms, real-time monitoring, behavioral analysis, and seamless integration all work together.
These features collectively provide a robust defense against fraud, ensuring your affiliate marketing efforts are safe and successful.
Here Are Practical Steps For Businesses Looking To Implement AI-Driven Fraud Detection Tools:
1. Assess Your Needs
- Identify Fraud Risks: Analyze your business’s specific fraud risks and challenges to determine the essential features of a fraud detection tool.
- Define Objectives: Set clear goals for what you want to achieve with the AI-driven tool (e.g., reduce chargebacks and improve transaction approval rates).
2. Select the Right AI-Driven Fraud Detection Tool
- Research Options: Compare available tools based on features, pricing, and target industries. Consider user reviews and case studies.
- Request Demos: Schedule demonstrations with shortlisted vendors to see the tools in action and assess their usability.
- Evaluate Scalability: Ensure the tool can scale with your business as it grows and adapts to changing fraud patterns.
3. Plan Integration
- Assess Existing Systems: Review your current systems (e.g., CRM, payment processors) to understand how the fraud detection tool will integrate.
- Create a Roadmap: Develop a detailed integration plan that includes timelines, responsibilities, and resource allocation.
- Engage IT Team: Involve your IT department early in the process to address technical challenges and ensure seamless integration.
4. Data Preparation
- Gather Historical Data: Collect historical transaction data to train the AI model and enhance its accuracy.
- Ensure Data Quality: Clean and organize data to avoid inaccuracies that could lead to false positives or negatives.
5. Train Staff
- Conduct Training Sessions: Provide comprehensive training for relevant staff on using the tool effectively and interpreting the essential features of its insights.
- Promote Collaboration: Foster communication between fraud detection teams, customer service, and IT to ensure a cohesive approach to fraud prevention.
- Develop Response Protocols: Create clear procedures for handling flagged transactions, including escalation processes for suspicious activities.
6. Monitor and Optimize
- Evaluate Performance: Regularly review the tool’s performance against your defined objectives, adjusting parameters as necessary.
- Gather Feedback: Solicit feedback from staff on tool usability and any issues encountered during implementation.
- Stay Updated: Keep abreast of the latest trends in fraud detection and continuously optimize your strategy based on new insights and evolving threats.
Here Are Some Future Trends In AI Fraud Detection To Consider:
1. Advancements in Technology In AI-Driven Fraud Detection Tools
- Enhanced Machine Learning Algorithms: Expect improvements in machine learning techniques, allowing for more accurate anomaly detection and pattern recognition. Advanced algorithms will better adapt to new fraud tactics in real-time.
- Natural Language Processing (NLP): The integration of NLP will enhance the ability to analyze textual data, such as customer interactions and transaction notes, helping to identify potential fraudulent behavior through sentiment analysis and contextual understanding.
- Blockchain Technology: The use of blockchain for secure and transparent transactions will grow, reducing fraud risks, especially in industries like finance and supply chain management. Smart contracts could automate fraud detection processes.
2. Regulatory Changes
- Increased Regulation: As fraud schemes evolve, regulatory bodies are likely to introduce stricter guidelines to ensure consumer protection and data privacy. Companies will need to adapt their fraud detection practices to comply with these changes.
- Global Standardization: There may be a move toward global standards for fraud detection and prevention, making it easier for businesses to implement consistent practices across different regions.
3. Evolving Fraud Tactics
- Sophisticated Cyber Attacks: Fraudsters are expected to use increasingly sophisticated tactics, such as AI-generated deepfakes or social engineering attacks, necessitating more advanced detection tools that can analyze behavior patterns beyond traditional indicators.
- Targeted Fraud Schemes: Using social media data and advanced analytics, fraud tactics will become more personalized and targeted. AI tools will need to incorporate behavioral analytics to detect anomalies in user behavior effectively.
4. Real-Time Detection and Response
- Instantaneous Fraud Alerts: The demand for real-time fraud detection will rise, leading to tools that provide immediate alerts and automated responses to suspicious activities, allowing businesses to act quickly and minimize losses.
- Adaptive Learning: AI systems will increasingly adopt adaptive learning capabilities, continuously evolving based on new data and emerging threats to improve detection accuracy over time.
5. Cross-Industry Collaboration
- Data Sharing Initiatives: Companies across industries may begin to collaborate and share data on fraud trends and threats, allowing for a more comprehensive understanding of fraudulent behaviors and improving overall detection capabilities.
- Public-Private Partnerships: Increased collaboration between government entities and private companies will facilitate the development of shared resources and tools to combat fraud more effectively.
6. Focus on Consumer Trust and Transparency
- Transparent Practices: Businesses will emphasize transparency in fraud detection processes to build consumer trust, clearly communicating how their data is protected and fraud is mitigated.
- Customer Education: Educating customers about potential fraud tactics and encouraging them to report suspicious activities will become a priority, fostering a proactive approach to fraud prevention.
7. Integration with Other Technologies
- IoT Integration: As the Internet of Things (IoT) expands, integrating fraud detection tools with IoT devices will help monitor transactions and user behavior across various platforms, enhancing detection capabilities.
- Use of Biometrics: The adoption of biometric authentication methods (fingerprints, facial recognition) will rise, providing an additional layer of security that AI-driven tools can analyze for fraud detection.
Here Are Some Valuable Resources For Readers Looking To Go Deeper Into AI-driven Fraud Detection:
Research Papers
- “Deep Learning for Fraud Detection: A Survey”
This paper provides a comprehensive overview of deep learning techniques applied in fraud detection, discussing various methodologies and their effectiveness.
Read it here - “Artificial Intelligence in Fraud Detection: A Survey”
This research examines the application of AI technologies in fraud detection across different sectors, highlighting trends and challenges.
Access the paper
Blogs
- Fraud Detection Blog by SAS
SAS offers insights and best practices in fraud detection, including case studies and the latest technology trends. Visit the SAS blog. - The Artificial Intelligence Blog
This blog features articles on AI advancements, including specific applications in fraud detection and prevention strategies.
Explore the AI blog
Webinars
- “The Future of Fraud Detection: AI and Machine Learning”
This webinar discusses the impact of AI and machine learning on fraud detection, showcasing practical applications and future trends. Watch the webinar. - “AI-Powered Fraud Prevention Strategies”
Hosted by industry experts, this webinar covers strategies for implementing AI-driven fraud detection tools in businesses. Register for the webinar.
Industry Reports
- “2024 Cybercrime Report” by Cybersecurity Ventures
This report offers insights into the state of cybercrime and fraud detection, detailing trends and future predictions. Download or read report. - “The State of Fraud Detection in 2024” by Experian
This annual report discusses current trends and challenges in fraud detection, providing actionable insights for businesses. Download or read report.
Pros and Cons of AI-Driven Fraud Detection Tools
Affiliate marketing has skyrocketed in popularity, offering businesses a cost-effective way to boost sales and gain brand exposure.
However, with the boom comes a downside—fraudulent activities. From click fraud to fake conversions, the dark side of affiliate marketing can erode trust and impact profitability.
When it comes to AI-driven fraud detection tools, there are some solid advantages and a handful of drawbacks.
Understanding these will help you decide whether jumping on the AI bandwagon is right for your affiliate marketing campaigns.
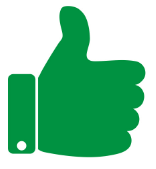
Pros:
High Accuracy:** AI tools excel at identifying fraud with high precision. They see patterns and anomalies humans might miss, drastically reducing fraudulent activities.
Real-Time Detection:** With instant alerts and real-time monitoring, these tools help you catch fraud as it happens, reducing potential damages.
Reduced Workload:** These tools take over the heavy lifting of data analysis, letting your team focus on strategy and creative work instead of combing through data manually.
Enhanced Data Security:** These tools help beef up your overall security posture by constantly monitoring and analyzing data, keeping your business and affiliate data safe.
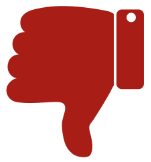
Cons:
High Implementation Costs:** Setting up AI-driven tools can be pricey. The initial investment might be steep, especially for smaller businesses.
Dependence on Data Quality:** AI is only as good as its fed data. Poor-quality or incomplete data can lead to inaccurate results, so you must ensure your data is top-notch.
Potential for False Positives:** Sometimes, AI’s overly cautious nature can flag legitimate activities as fraud, leading to unnecessary disruptions.
Privacy Concerns:** The extensive data collection involved can raise privacy issues. It’s crucial to handle all user data responsibly and transparently.
Balancing these pros and cons will give you a clearer picture of whether AI-driven fraud detection tools fit your needs. Always weigh the benefits of improved accuracy and security against the costs and potential challenges.
Here Are Some Real-Life Case Studies And Success Stories Featuring Individuals And Companies That Have Successfully Utilized AI-driven Fraud Detection Tools:
Mark Johnson, Digital Marketing Manager at XYZ Corp
Faced with rising click fraud impacting their affiliate marketing campaigns, Mark implemented an AI-driven fraud detection tool called FraudShield. Within three months, the tool helped identify and block over 1,000 fraudulent clicks, resulting in a 30% increase in legitimate conversions and significant cost savings for the company.
Sarah Thompson, E-commerce Director at TrendyGoods:
Sarah’s team struggled with high return rates due to fraudulent transactions. By adopting Sift, an AI-based fraud detection platform, they could analyze transaction patterns in real-time. As a result, they reduced fraud-related losses by 40% within the first year and improved their customer satisfaction ratings.
David Lee, Founder of TravelSmart:
David launched an AI-powered tool called Forter to combat identity theft in his travel booking platform. The tool used machine learning to assess user behavior and transaction data. After implementation, TravelSmart saw a 50% decrease in fraudulent bookings, allowing the company to expand its services without fearing fraud-related revenue loss.
Emily Carter, Head of Affiliate Marketing at ClickBoost:
Emily’s team faced affiliate impersonation challenges, leading to significant revenue loss. After integrating Riskified, an AI-driven solution, they were able to detect and block fraudulent affiliates effectively. This action resulted in a 25% increase in overall affiliate revenue within six months, as only legitimate affiliates were retained.
Michael Nguyen, Operations Manager at Fintech Solutions:
Michael’s company adopted Kount, an AI fraud detection tool, to protect against payment fraud. The platform’s predictive analytics helped them identify high-risk transactions, leading to a 60% reduction in chargebacks. This success improved their bottom line and enhanced their reputation in the fintech industry.
Expert Insights: Leaders Have Praised AI-Driven Tools.
Expert Insights: Industry leaders have praised AI-driven tools for their accuracy and efficiency.
Marketing experts believe that these tools have set a new standard in fraud detection, making affiliate marketing more secure and reliable.
They emphasize that the initial investment in AI pays off through long-term gains and reduced manual efforts.
Lessons Learned: The key takeaways from these success stories include the importance of continuous monitoring, the need for high-quality data, and the benefits of quick decision-making.
Businesses that leverage AI see substantial improvements in fraud detection and overall campaign performance, making these tools indispensable in today’s affiliate marketing landscape.
Q&A Section and Conclusion
Common Q&A About AI-Driven Fraud Detection Tools:
Q1: What are AI-driven fraud detection tools?
A1: AI-driven fraud detection tools are software solutions that utilize artificial intelligence and machine learning to identify and prevent fraudulent activities. They analyze vast amounts of data in real-time, detecting anomalies and patterns indicative of fraud.
Q2: How do these tools work?
A2: These tools use algorithms to process historical and real-time data, learning from past fraud patterns. They monitor user behavior, transaction history, and other data points to flag suspicious activities automatically.
Q3: What types of fraud can these tools detect?
A3: AI-driven fraud detection tools can identify various types of fraud, including click fraud, affiliate impersonation, account takeover, payment fraud, and transaction anomalies.
Q4: What are the benefits of using AI-driven fraud detection tools?
A4: The benefits include real-time monitoring, improved accuracy in detecting fraud, reduced false positives, enhanced security, and the ability to adapt to new fraud tactics over time.
Q5: Are there any drawbacks to using these tools?
A5: Potential drawbacks include high implementation costs, the need for ongoing training and maintenance, reliance on data quality, and the possibility of false negatives or positives if the algorithms are not finely tuned.
Q6: How can businesses choose the right AI-driven fraud detection tool?
A6: Businesses should assess their specific needs, consider the types of fraud they face, evaluate the tool’s features, review case studies, and seek demos or trials to understand how well the solution integrates with their existing systems.
Q7: Can small businesses benefit from AI-driven fraud detection tools?
A7: Absolutely! These tools can significantly benefit small businesses, as they help protect against fraud losses. Many solutions are scalable and can be tailored to fit the budget and needs of smaller enterprises.
Q8: How can AI-driven fraud detection tools improve customer trust?
A8: These tools foster a safer online environment by effectively reducing fraudulent transactions and protecting customer data. This enhances customer trust, as clients feel more secure in their transactions with the business.
Q9: What industries can benefit the most from AI-driven fraud detection tools?
A9: Industries such as e-commerce, finance, travel, gaming, and affiliate marketing are particularly vulnerable to fraud and can greatly benefit from implementing these advanced detection tools.
Q10: What does the future hold for AI-driven fraud detection?
A10: The future of AI-driven fraud detection looks promising, with advancements in machine learning and data analytics. As fraud tactics evolve, these tools will likely become more sophisticated, offering better detection capabilities and enhanced security features.
Conclusion
As I conclude this article, I have to say that implementing AI-driven fraud detection tools is not just an option but a necessity.
Lets face it, AI is taking over wheather you like it or not, big companies are using it, why not you as an affiliate marketer?
There is an AI for everything and we can certainly used it to our advantage. As affiliate/Online meaketers you have, AI for SEO, AI ChatBot for customer support, AI for Analytics and so many more AI platforms.
As we’ve explored throughout this article, these tools provide powerful, real-time capabilities to identify and mitigate fraud, safeguard investments, and foster trust among partners.
While initial costs and data quality may be challenging, the benefits outweigh the drawbacks.
Businesses that embrace these technologies are better equipped to protect their revenues, improve their ROI, and create more reliable relationships with their affiliates.
We’d love to hear your thoughts!
- Have you experienced fraud in your affiliate marketing efforts?
- What tools are you considering, or have you already integrated AI solutions into your strategy?
Share your experiences in the comments below, and let’s discuss how we can collectively combat fraud in this dynamic industry.
Thanks for such a great article!! It’s amazing how AI is transforming affiliate marketing, especially with fraud detection. The breakdown of the different tools and their capabilities was super helpful—definitely some new insights there! I appreciate the detailed pros and cons too; it’s great to see a balanced view on how AI can boost security while also acknowledging the potential challenges. Can’t wait to see how these tools evolve and continue to shape the future of affiliate marketing. Thanks again for sharing!
Thank you for the kind words! I’m glad you found the article helpful. It’s exciting to see how AI is revolutionizing affiliate marketing, especially in fraud detection.
The future looks promising, and I’m eager to see how these tools continue to evolve!
Fraud is one of the most challenging issues for many institutions, including finance and healthcare. It’s true that they have to get the right equipment to protect their assets and their clients. personally, I didn’t know that so many solutions existed! I have seen the fraud solutions for the bank I worked for, but I don’t remember that they were AI powered!
You’re spot on—fraud is a major issue, and AI-powered solutions are now leading detection and prevention.
It’s impressive how far technology has come, and many institutions are starting to adopt these tools for better protection. If you haven’t seen AI in action yet, it’s worth checking out the latest advancements!
Thank you for your comments.